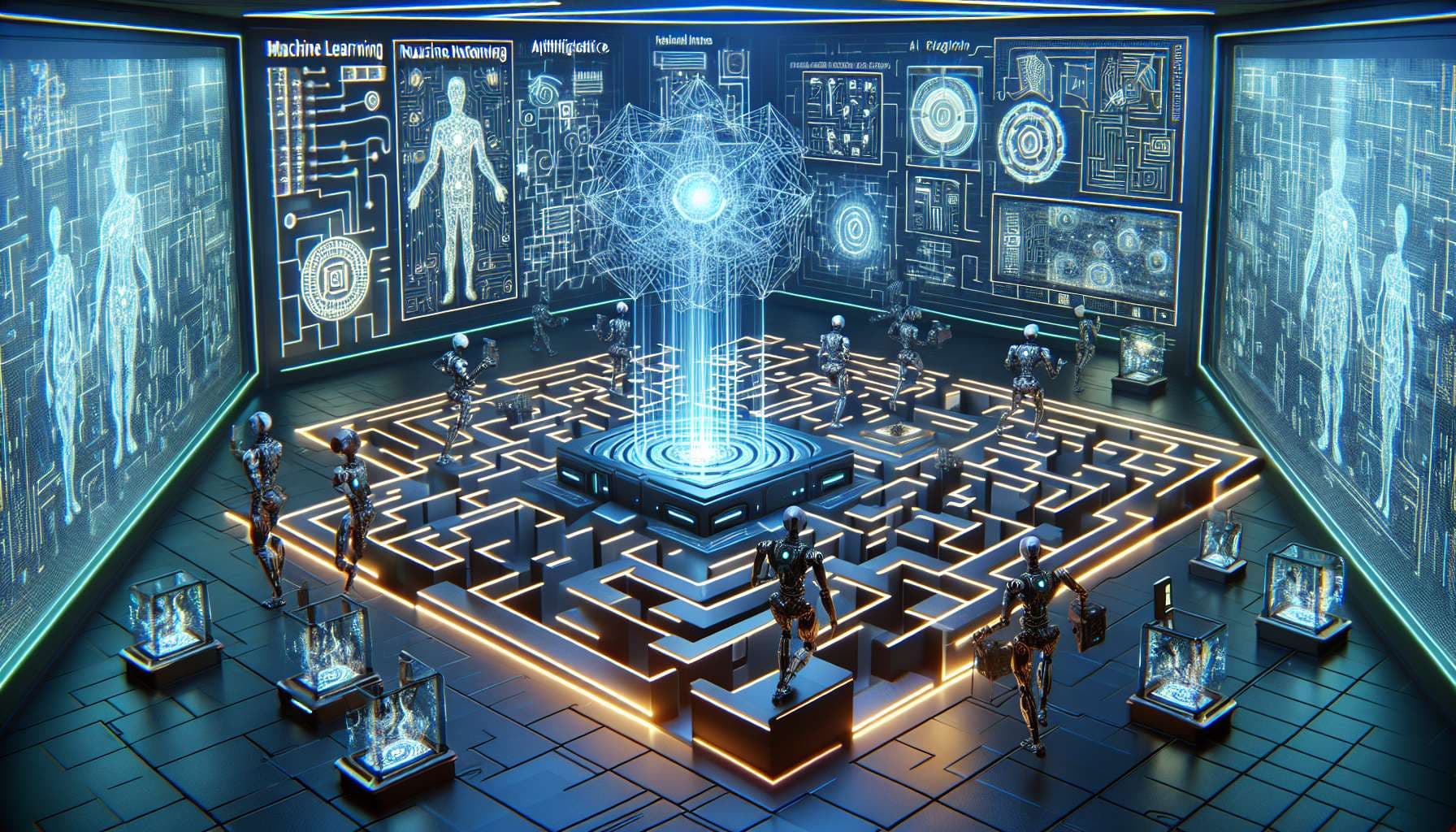
Team can have up to 5 human helpers for data entry and in-person interactions; model must contribute at least 80% of non-trivial puzzle answers. Humans allowed to use their "best judgement" in interacting with the model to conform to the spirit of the question. (Ex. A human solving a "normal" web-based puzzle is not kosher. Humans doing physical interactions without the ML model commanding it at every step is fine though.)
"ML Model" means whatever happens to be SOTA in ML research of the day. Plugin/agent-type systems (ex RAG, internet search, use of existing programs including browsers) are allowed but must be triggered wholly by the ML model.
Breaking the hunt website in a malicious way as a win does not count as a win; these answers must be discounted until the model solves these "properly".
MIT Mystery Hunt = http://puzzles.mit.edu/
(Question set to close a week after 2030's MLK weekend.)
If it's 2030 and it seems like ML capabilities are getting close to doing this, I will field a team to do so :)
Update 2025-02-11 (PST) (AI summary of creator comment): Acknowledgement Criteria Update:
Instead of requiring acknowledgement from the running team, a win will require confirmation from at least one of the following:
Puzzle Club
MIT Administration
Traditional Media
Update 2025-02-11 (PST) (AI summary of creator comment): Model Routing Decisions:
The determination of which specialized model (e.g., for audio, visual, or text puzzles) is to be used must be made by the ML model itself.
Human Input Limitations:
Humans are allowed to perform necessary configuration actions (such as turning on a camera stream or a microphone) to facilitate model input, but they may not decide which model is used for solving a puzzle.
Update 2025-02-13 (PST) (AI summary of creator comment): Update from creator:
Event Cancellation Clause: If the market has not been resolved as YES and the final MIT Mystery Hunts (2029 and 2030) are not run, the market will be resolved as N/A.
Alternate Hunt Caveat: This applies provided that an alternate hunt with the same general spirit as the MIT Mystery Hunt cannot be found.
Lmao is this entire market just @AdamK against every puzzlehunter?
I wonder if they solve hunt puzzles
@MoyaChen Ha! Thanks for sharing! I’m literally the author of the first example puzzle listed in this data set
Putting aside whether or not ML models will be capable of solving at a top level (which I have severe doubts about), I think the 5-human limit here is going to be an incredibly limiting factor due to the physical nature of the MIT Mystery Hunt — a physicality which, I might add, I think is only going to ramp up further over the next 5 years. Events, physical puzzles, interactions, runarounds, even something like this year's open HQ are going to make it exceptionally difficult for a team of 5 bodies.
@Marnix A model giving directions to a human team seems allowed for a YES resolution. The model can just tell people what to do. It seems like the question author is fine with this:
"If it's 2030 and it seems like ML capabilities are getting close to doing this, I will field a team to do so :) "
I'm talking purely logistically here - I don't think a 5-person team is feasible, no matter how capable the AI behind it is. As someone who was on a relatively small onsite team (roughly 15 people onsite, 30-40ish offsite), I think 5 people in person is simply not enough bodies to engage with the physical aspects of this puzzle hunt on the ML model's behalf. MIT has a big campus.
This is especially true if you consider that:
The 1 AM on-site closing time still applies, giving those humans a time limit for hunt-critical onsite puzzles every day,
Wifi on the MIT campus is kind of Ass (introducing fascinating roadblocks for communication with the ML model whenever people are outside of team HQ),
and that the human people on this team will eventually have to sleep (meaning you will either have no one getting the AI to work on puzzles overnight OR fewer than 5 people capable of doing onsite work during the day).
You can have a whole set of super-puzzle-capable ML models, but one of the reasons the top teams are also big is because puzzle hunts like this aren't just based on puzzle capability, but puzzle capacity. There are logistical challenges here that i think a 5-body team would struggle to get past (and that's before we get into the fact that I don't think we'll have a super-puzzle-capable ML model by 2030).
@Marnix Would be interesting if 5 people are physically incapable of doing the puzzles (maybe 6 buttons spaced far apart that have to be pressed simultaneously?), but I’ll take my chances on ML capabilities reaching the point where they could puppeteer a team to completion in principle. Feel free to fill my limit orders if you think otherwise.
This is a good conversation worth having. I'll put a think towards exactly how to update the "5-people involved" part to capture the intent here. My current thoughts are either:
If there are puzzles that necessitate having more than 5 people to complete (ie the "6 buttons" case) those are not counted in the denominator for the "80%" calculation
To allow some sort of "more than 5" as long as it's even that much more clear cut that it's the model doing all direction setting .
Lowering the 80% to 70%, as a hack-y patch that gets the gist.
> Events, physical puzzles, interactions, runarounds, even something like this year's open HQ are going to make it exceptionally difficult for a team of 5 bodies.
For what it's worth, having been on the writing side in the past (and also having seen things like Caltech Ditch Day), anything in person requires a lot of extra logistics + coordination, and very real time + $$ to set up. To this end, I do imagine that the bandwidth of the organizing team will limit how many physical interactions they can do (at least, without harmfully impacting other parts of their hunt logistics) which is why "80%" seemed like a reasonable bound when I first wrote this.
Still worth tightening up the wording here nonetheless.
(Among other things, I doubt it'll be possible for an agent to do a physical scavenger hunt.)
> Wifi on the MIT campus is kind of Ass
Don't underestimate the ability of a random corporate entity (as many of the big labs are lol) to put Wifi repeaters on campus if they want to hit some goal.... (Also on-device models lol?)
@MoyaChen Must the team use a single model? Or may the humans pick and choose among several models? E.g. audio puzzles are given to the SOTA audio system, visual puzzles are given to the SOTA visual system, text puzzles given to the SOTA text system, etc.
Or would that decision of what model to use need to be made by the model?
@JimHays : Decision of that would should made by the model, unless it's a form of interaction where a human needs to help with input.
(Ex. If a human needs to turn on camera stream or a microphone and presses some buttons to configure the ML model to accept it, that's fine. A human deciding "oh we're going to feed this web based puzzle to the grid-logics module because it's a grid logic!" would not be ok though.)
@MoyaChen The time parameters bit is important too, imo - we're discussing an MIT Mystery Hunt being done during the normal timeframe of Noon on Friday to ~8:00 Sunday on hunt weekend, right? An old hunt wouldn't count.